Posted 02/4/13
What are the most important predictors of ecological processes? And what is the best way to pick them out? These are answers to which Nick Keuler, the SILVIS statistics specialist has innovative answers to: instead of stepwise regression, we should use multi-facetted approaches to determine variable importance in ecological models. Here, Nick explains the hierarchical partitioning and the best subsets regression methods, which can be used to answer questions ranging from invasive species to satellite image texture.
It is always a challenge to transform the real world into a mathematical or statistical model, which you can then reliably apply to the world around you. When you have something you want to predict, and a list of potential predictors, how do you decide which variables are important? Picking the important variables is no trivial task: Nick points out that often times, scientists use simplistic methods for picking their predictors, for example, assuming variables that end up in a ‘final’ model based on a stepwise regression are the ones that are important. This might yield erroneous or incomplete results. Thankfully, here in the SILVIS lab Nick helps us understand methods that reduce the risk of missing any predictor that might be important in an ecological model. Instead of simply focusing on the practical significance (which variable causes the biggest change in the response) or the statistical significance (p-value) of a variable, Nick is trying to wean us off of stepwise regression and instead advocates the use of a multi-facetted approach to determining variable importance in ecological models: the hierarchical partitioning method and the best subsets regression method. These sound like complex terms, but Nick explains: ‘In hierarchical partitioning, you can think of the total variability in the response variable as a big bucket of water. Explained variability is like water removed from the bucket, and each variable added to the model will remove some water. Using hierarchical partitioning, we can attribute each drop of water to one of the variables, and the more important variables are those that remove a larger proportion of the water. There will always be some water left in the bucket, representing the variability that is not explained by any variable, which we call the residuals.’The best subsets regression method essentially fits all models of all possible sizes, and uses some criterion, such as R^2, to sort the models from ‘best’ to ‘worst.’ Variables that tend to appear in the best models are the ones that are most important. The two methods often yield comparable results, but best subsets can generally be used on data sets with larger numbers of variables. Fitting every possible model is computer intensive, so these methods have only come into vogue as computers get faster. ‘With these methods, we make sure we do not ignore any relevant variables and don’t achieve misleading conclusions about the importance of the variables, especially when some of the predictors may be correlated.’ Nick explains. Then he smiles and adds, ‘If we can figure out which variables are important, then we can focus our limited time and money on changing those things in ways that move our responses in beneficial ways’. With Nick’s help, these methods are applied across SILVS on research ranging from invasive plants in the Baraboo Hills to agriculture field image texture in Eastern Europe.
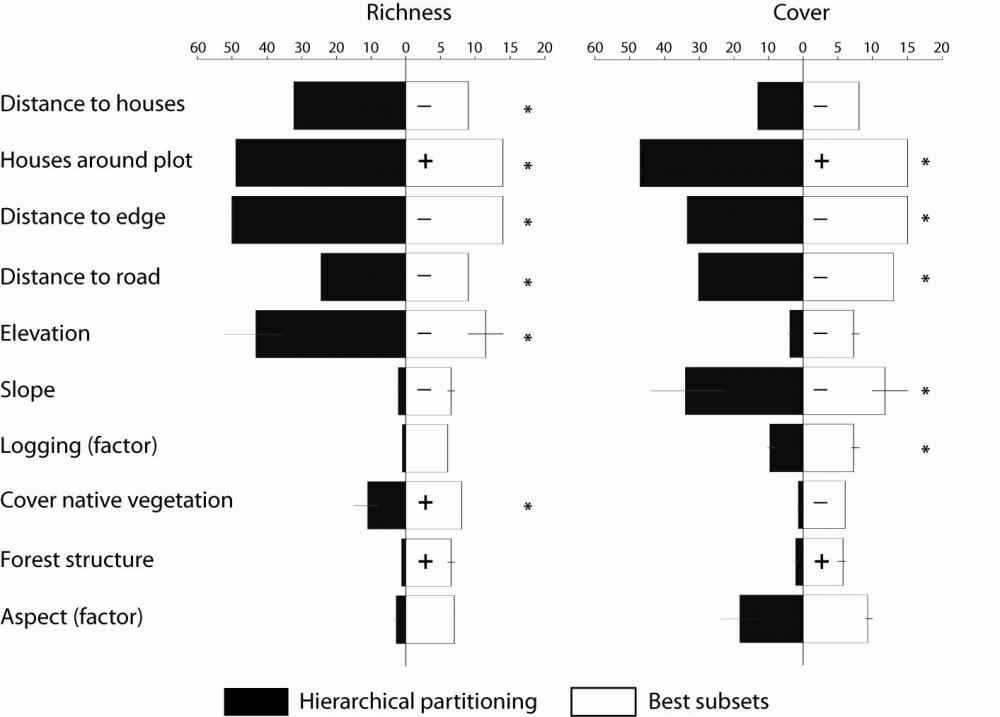
“
Story by Catalina Munteanu