Posted 04/2/13
Monitoring land cover change through time is important for research projects trying to understand change at local and global scales. Satellite imagery provides data over broad extents that capture information on land cover features such as vegetation types and habitat structure. In order to accurately capture land cover as it appears on the ground, imagery pixels need to be 'clean' (i.e., not contain clouds or their shadows). Nonetheless, much of the earth is often covered in clouds, posing difficulties for scientists studying land change from satellite imagery. Silvis lab researcher Glen Aronson is tackling this issue in his research to track deforestation in Mexico over the past two decades.
Many ecologists desire to understand how land cover changes over time. A popular approach is to use satellite imagery, which provides data over broad spatial and temporal extents. SILVIS Lab researcher Glen Aronson is working to understand deforestation trends throughout Mexico over the past two decades in order to evaluate the effectiveness of Mexico’s national Payment for Ecosystem Services Program. Surely a study like this could not be carried out with data collected by researchers surveying Mexico’s forests on foot. Along with principal investigator Jennifer Alix-Garcia, and SILVIS researchers Volker Radeloff and Carlos Ramirez-Reyes, Glen analyzes imagery from the Landsat series of satellites, with each satellite image covering an area of 185 km x 185 km. However, a persistent difficulty with this approach to land change study is finding images that are completely cloud free. Especially in tropical regions of the world, cloud cover is a constant problem making large sections of satellite images unusable in land cover change studies. ‘In southern Mexico especially, there is near-constant cloud cover which makes it very difficult for us to see deforestation patterns in the satellite imagery’, notes Glen. Unfortunately, most forest change detection algorithms can only be applied to cloud free images because clouds and cloud shadows in images can be incorrectly interpreted as evidence of forest change. In order to tackle this problem, Aronson has been experimenting with cloud detection algorithms designed to automatically locate clouds and cloud shadows within images. Once Glen has isolated the cloudy portions of an image, he substitutes the cloudy pixels with clear pixels from other images of the same area taken within the same season as the base image. ‘By separating cloudy pixels from clear pixels, these algorithms allow us to compare cloud-free composite images through time,’ says Glen. He has used a number of cloud masking algorithms but has found the Fmask algorithm developed by researchers at Boston University to be the most accurate and useful. This particular algorithm differs from others in that it not only identifies clouds based upon their bright spectral characteristics unique thermal signatures, but also uses a segmentation algorithm to determine the shapes of cloud objects. This feature makes the algorithm especially good at detecting cloud shadows, which typically have the same shape as their associated clouds.
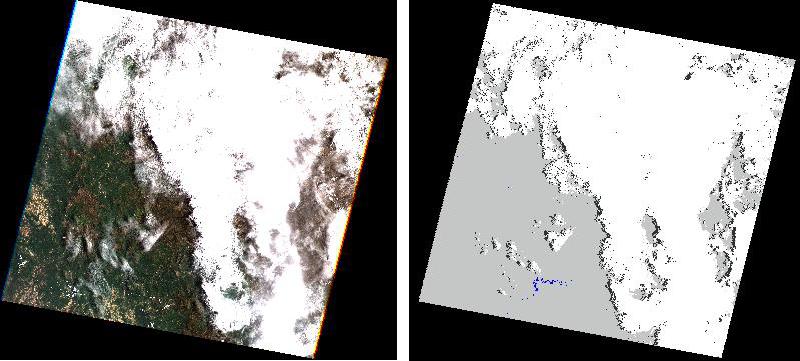
Because Glen’s research involves tracking forest change through thousands of Landsat images, his work would be far too labor intensive without the help of automated cloud masking algorithms like Fmask. Given all the time saved by automated cloud masking algorithms, he has ample time to work on an even more challenging task: figuring out how to automate forest change detection.”
Story by Eric Wood